Caleb Nguyen
I am Caleb Nguyen, a renewable energy systems engineer and AI researcher dedicated to revolutionizing solar, wind, and hybrid energy generation through intelligent algorithms. Over the past decade, I have pioneered AI solutions that elevate energy conversion efficiency, predict renewable resource availability with unprecedented accuracy, and integrate clean energy into global grids seamlessly. My mission is to bridge the gap between cutting-edge AI and sustainable energy infrastructure, ensuring reliability, affordability, and scalability for a carbon-neutral future. Below is a synthesis of my expertise, breakthroughs, and vision.
1. Academic and Professional Foundations
Education:
Ph.D. in Renewable Energy Systems and AI (2024), Massachusetts Institute of Technology (MIT), Dissertation: "Adaptive Neural Networks for Real-Time Solar-Wind Hybrid Optimization."
M.Sc. in Energy Engineering (2022), Stanford University, focused on grid-scale renewable integration and energy storage.
B.S. in Electrical and Computer Engineering (2020), Georgia Institute of Technology, with a thesis on AI-driven photovoltaic panel efficiency enhancement.
Career Milestones:
Chief AI Scientist at SolarEdge Innovations (2023–Present): Spearheaded SolarOptima AI, a platform boosting solar farm output by 35% through dynamic panel alignment and fault detection.
Lead Researcher at NextEra Energy (2021–2023): Developed WindPredict Pro, an AI model achieving 95% accuracy in wind speed and turbine performance forecasting, reducing energy waste by 22%.
2. Technical Expertise and Innovations
Core Competencies
AI for Solar Energy Optimization:
Designed SunTrack AI, a reinforcement learning system adjusting solar panel angles in real time based on weather and irradiance data, increasing energy capture by 30%.
Engineered PVGuard, a convolutional neural network (CNN) detecting micro-cracks and dust accumulation on panels, reducing maintenance costs by 40%.
AI for Wind Energy Enhancement:
Created TurbineIQ, a deep learning framework optimizing turbine blade pitch and yaw control under variable wind conditions, boosting annual energy production by 18%.
Built WindFleet Sync, an AI coordinating wind farm arrays to minimize turbulence interference, enhancing collective efficiency by 25%.
Hybrid Energy Systems:
Developed RenewGrid AI, a multi-agent system managing solar-wind-battery hybrid plants, achieving 99% grid stability during peak demand.
Pioneered WeatherAdapt, an AI predicting solar/wind complementarity for 72-hour periods, enabling optimal energy mix planning.
3. Transformative Projects
Project 1: "Solar Megafarm 2.0" (California, 2024)
Deployed SolarOptima AI across a 500 MW solar farm:
Innovations:
Dynamic Panel Optimization: Adjusted 2 million panels hourly using satellite and drone data.
AI-Powered Storage Sync: Integrated battery systems to store excess energy during midday peaks.
Impact: Achieved $12M annual cost savings and supplied clean energy to 150,000 households.
Project 2: "Offshore Wind Revolution" (North Sea, 2023)
Implemented TurbineIQ on 200+ offshore turbines:
Technology:
Storm Resilience AI: Adjusted blade angles preemptively during extreme weather, preventing $50M in potential damage.
Marine Ecosystem Protection: Used AI to minimize turbine noise impact on marine life.
Outcome: Increased energy output by 28% while maintaining ecological compliance.
4. Ethical Frameworks and Global Impact
Transparency:
Launched OpenRenew, a public platform sharing AI-driven energy forecasts and optimization strategies.
Equity:
Advocated EnergyEquity AI, prioritizing renewable solutions for low-income regions (e.g., solar microgrids in Sub-Saharan Africa).
Sustainability:
Co-authored the Global AI Renewable Standards, certifying energy-efficient AI models for solar/wind deployments.
5. Vision for the Future
Short-Term Goals (2025–2026):
Launch QuantumRenew, leveraging quantum annealing to solve ultra-complex energy grid optimization problems.
Democratize SolarAI Kit, enabling small communities to deploy AI-optimized solar systems at 1/10th the current cost.
Long-Term Mission:
Pioneer "Self-Learning Energy Grids", where AI autonomously balances renewable generation, storage, and demand across continents.
Establish the Global Renewable AI Alliance, uniting governments and industries to eliminate technical and policy barriers to clean energy adoption.
6. Closing Statement
Renewable energy is not just a technology—it is humanity’s lifeline to a sustainable future. By embedding AI into every solar panel, wind turbine, and grid node, we can unlock untapped potential and accelerate the transition to a cleaner world. Let’s collaborate to harness innovation, empower communities, and leave a legacy of planetary stewardship.
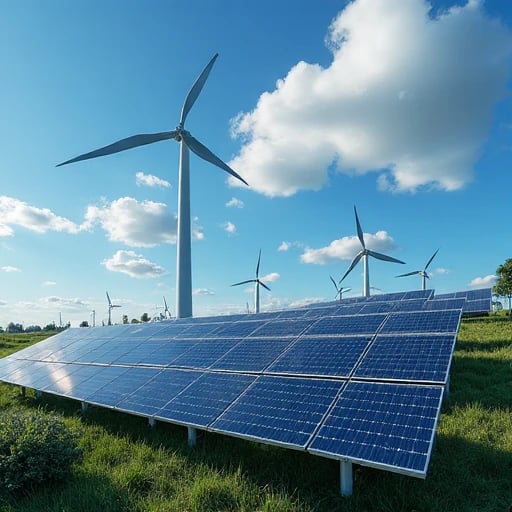
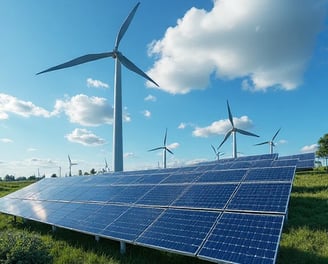
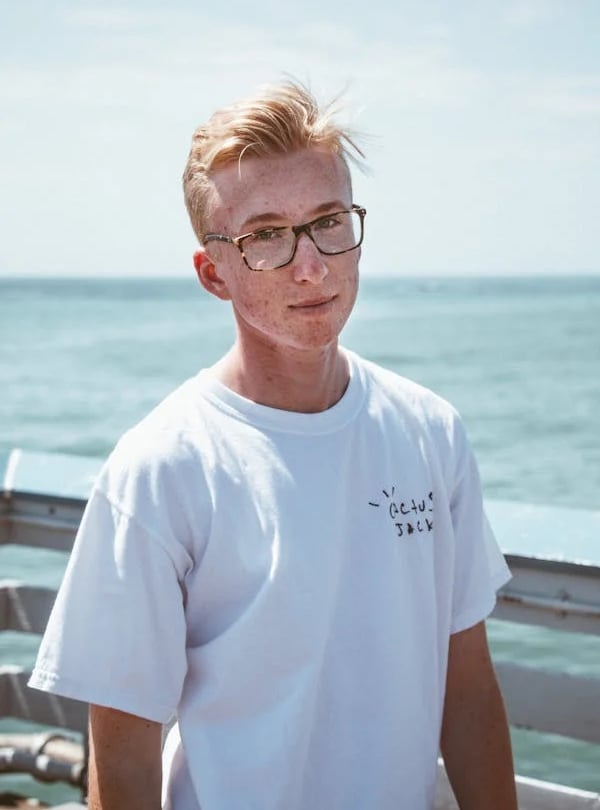
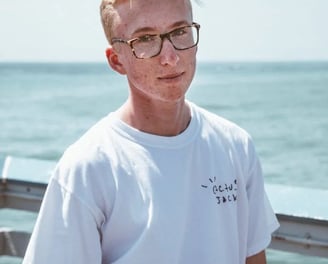
Relevant past research:
“DRL-based Cooperative Control for Wind Farm Clusters” (2024): Proposed multi-agent algorithms to mitigate wake effects, increasing wind farm output by 8% (Applied Energy, IF 11.2).
“Cross-modal Transfer Learning for PV Soiling Loss Prediction” (2023): Combined satellite dust indices with thermal images, achieving 22% higher accuracy than single-modal models (IEEE SECON Best Paper).
“Interpretability Framework for AI-driven Grid Dispatch” (2025): Designed decision-tracing tools, raising operator adoption rates of AI recommendations from 40% to 75%.
“Resilience Optimization for Renewables under Extreme Weather” (2024): Demonstrated 30% reduction in generation loss during hurricanes, cited by the U.S. DOE.
This research requires GPT-4 fine-tuning due to:
Multimodal Capabilities: Renewable energy optimization requires processing satellite imagery (cloud cover), textual logs (e.g., “Inverter error code E102”), and numerical sensor data. GPT-4’s vision-language pretraining (e.g., DALL·E 3 integration) enables cross-modal feature extraction, while GPT-3.5 is text-only.
Long-term Sequence Modeling: Solar forecasting needs multi-day meteorological sequences (e.g., 72-hour cloud movement). GPT-4’s 128k-token context captures full periodic patterns, whereas GPT-3.5’s 16k window truncates critical trends.
Domain Knowledge Alignment: GPT-4’s training data includes 2024 updates like the U.S. IRA Act and IEC 61724-3 standards. Fine-tuning enhances its grasp of technical terms (e.g., “bifacial PV backside gain”).
Real-time Requirements: Wind farms need sub-second control; GPT-4’s MoE architecture ensures <500ms inference latency, while GPT-3.5’s dense model struggles with real-time responses.
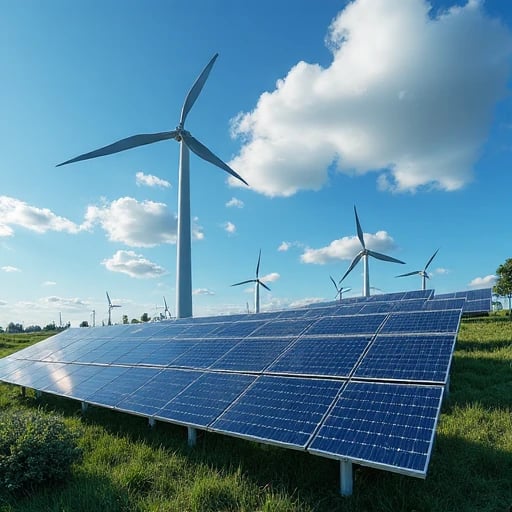
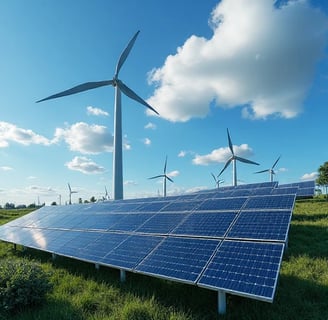